The Power of Machine Learning: Unveiling the Future of Technology
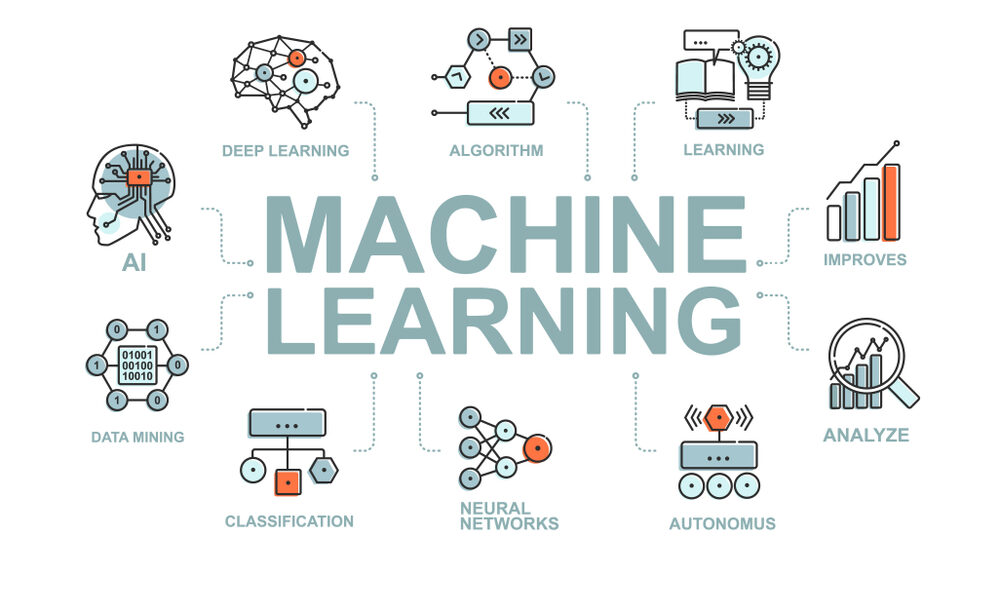
Introduction: Embracing the Era of Machine Learning
In an era marked by rapid technological advancements, machine learning has emerged as a transformative force, reshaping industries and revolutionizing the way we interact with technology. This article delves into the intricate world of machine learning, unraveling its complexities, applications, challenges, and future prospects. As we journey through this landscape, we aim to provide a comprehensive understanding of how machine learning is poised to shape our future.
Understanding Machine Learning: A Deeper Dive
Breaking Down Machine Learning Concepts
At its core, machine learning is a subset of artificial intelligence that equips computers with the ability to learn from data and improve their performance over time without being explicitly programmed. Supervised learning, unsupervised learning, and reinforcement learning stand as the three primary categories under which machine learning algorithms operate. In supervised learning, the algorithm learns from labeled data, while unsupervised learning identifies patterns in unlabeled data. Reinforcement learning involves training models to make sequences of decisions through trial and error.
Types of Machine Learning Algorithms
Machine learning algorithms encompass a wide spectrum, including classification, regression, clustering, and dimensionality reduction. In classification, models categorize data points into predefined classes, while regression predicts continuous values. Clustering groups similar data points together, and dimensionality reduction reduces the number of features in a dataset, simplifying complex problems.
Applications of Machine Learning Across Industries
Healthcare: Revolutionizing Medical Diagnostics
Machine learning is making significant strides in healthcare by enhancing diagnostic accuracy and enabling personalized treatment plans. From image recognition for early disease detection to predictive analytics for patient outcomes, these algorithms are paving the way for more efficient and precise medical interventions.
Finance: Enhancing Predictive Analytics
In the financial sector, machine learning is transforming how institutions analyze vast datasets to predict market trends, assess risks, and optimize investment strategies. Fraud detection algorithms, powered by machine learning, are becoming more adept at identifying anomalous patterns and safeguarding financial transactions.
E-commerce: Personalizing Shopping Experiences
E-commerce platforms leverage machine learning to create personalized shopping experiences for consumers. Recommendation engines analyze user behavior and preferences to suggest products, ultimately boosting customer engagement and sales.
Automotive: Pioneering Self-Driving Cars
Machine learning is the driving force behind the development of self-driving cars. These vehicles rely on complex algorithms to process real-time data from sensors and make split-second decisions, revolutionizing the future of transportation.
How Machine Learning Works: Key Components and Processes

Data Collection and Preprocessing
The foundation of effective machine learning lies in high-quality data. Data collection involves gathering relevant information, which is then preprocessed to remove noise, handle missing values, and normalize features. Clean, well-structured data is crucial for training accurate models.
Training and Testing Models
The training phase involves feeding labeled data into the algorithm, allowing it to learn and establish patterns. Testing data assesses the model’s performance, enabling adjustments to enhance accuracy. This iterative process continues until the model achieves the desired level of performance.
Iterative Refinement: Fine-Tuning the Model
Machine learning models require constant refinement. By adjusting parameters, incorporating more data, or altering algorithms, developers iteratively improve the model’s accuracy and effectiveness. This ongoing refinement ensures optimal performance in dynamic environments.
Overcoming Challenges in Machine Learning Implementation

Data Privacy and Security Concerns
The use of sensitive data in machine learning raises privacy and security concerns. Striking a balance between data utilization and individual privacy is imperative to maintain trust and comply with regulations.
Bias and Fairness in Machine Learning
Machine learning algorithms can inadvertently perpetuate biases present in training data. Ensuring fairness and mitigating biases require careful curation of diverse and representative datasets, coupled with algorithmic techniques that promote equitable outcomes.
Interpretability: Understanding Black Box Models
The inherent complexity of certain machine learning models, often referred to as black box models, raises challenges in understanding how decisions are made. Developing methods to interpret and explain these models is critical for fostering transparency and user trust.
Frequently Asked Questions About Machine Learning
- What is the role of labeled data in machine learning? Labeled data serves as the foundation for training supervised learning models. It consists of input-output pairs that enable algorithms to learn patterns and make predictions.
- Can machine learning models learn from unstructured data? Yes, machine learning models can extract insights from unstructured data like text, images, and audio. Techniques like natural language processing and computer vision enable the analysis of diverse data types.
- How does machine learning differ from traditional programming? Traditional programming involves explicit instructions, while machine learning learns patterns from data. It excels in tasks with complex patterns or large datasets, where manual programming is impractical.
- What are some popular machine learning frameworks? Common machine learning frameworks include TensorFlow, PyTorch, and scikit-learn. These libraries provide tools for building, training, and deploying machine learning models.
- Is machine learning the same as artificial intelligence? Machine learning is a subset of artificial intelligence. While AI encompasses a broader range of technologies that simulate human intelligence, machine learning focuses specifically on algorithms that learn from data.
The Future of Machine Learning: Trends and Innovations
Reinforcement Learning: Advancements in AI Training
Reinforcement learning is gaining traction, with applications in robotics, gaming, and autonomous systems. It involves training agents to make sequential decisions by maximizing rewards, paving the way for more capable AI systems.
Federated Learning: Collaborative and Secure Model Training
Federated learning enables multiple devices to collaboratively train a shared model while keeping data localized. This approach enhances privacy and security, making it ideal for applications in healthcare and finance.
Explainable AI: Bridging the Interpretability Gap
The quest for interpretable machine learning models continues. Explainable AI aims to make complex models more transparent, enabling users to understand and trust the decisions made by AI systems.
Conclusion: Embracing a Transformed Technological Landscape
As machine learning continues to permeate diverse industries, its transformative potential is undeniable. From healthcare to finance, from e-commerce to transportation, machine learning is reshaping the way we interact with technology and opening new avenues for innovation. By understanding its concepts, applications, challenges, and future trends, we position ourselves to harness the power of machine learning and shape a future where intelligent systems drive progress and possibility.